Torque Clustering: Autonomous AI is Approaching
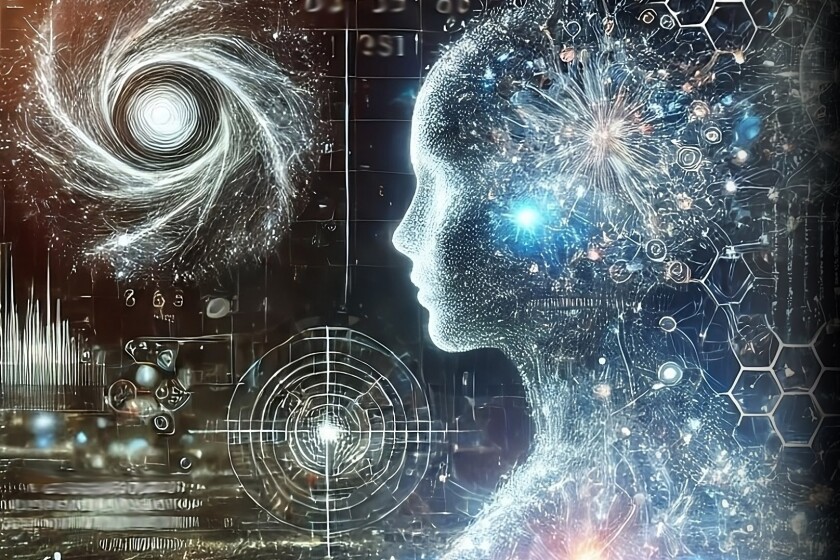
Image generated by DALL-E
Every ten minutes or so, another article about a “groundbreaking” AI breakthrough pops up on my screen. A new method, a new capability, billions invested here, AI agents there. The cycle has been relentless for the past year, accelerating by the day. Today was no exception.
Every ten minutes or so, another article about a “groundbreaking” AI breakthrough pops up on my screen. A new method, a new capability, billions invested here, AI agents there. The cycle has been relentless for the past year, accelerating by the day. Today was no exception.
When I gaze at the sea across the street, the horizon appears to be around four miles (six km) away—where the Earth’s curvature takes effect. That’s not exactly a great distance. Is “fully” autonomous AI really that close?
I have my doubts, but the latest AI breakthrough from the University of Technology Sydney might just make me reconsider.
University of Technology Sydney unveils Torque Clustering, an AI training method inspired by merging galaxies
Researchers at the University of Technology Sydney have introduced a novel AI training method called Torque Clustering. Inspired by the gravitational dynamics of merging galaxies, this approach is designed to efficiently and autonomously process vast datasets without human intervention or predefined parameters—marking a stark contrast to traditional AI clustering techniques.
According to Prof. Chin-Teng Lin, the process mirrors natural learning, where “animals learn by observing, exploring, and interacting with their environment, without explicit instructions.”
Think of clustering like a party. You scan the room and notice groups of people gathered based on shared interests—sports, BBQ, gardening—while one person stands alone in the corner. This is the simplest way to understand how clustering works.
When AI analyzes a dataset, it sorts similar data into groups or patterns for efficient processing. Several clustering methods exist, with K-Means, DBSCAN, and Hierarchical Clustering being the most common. Each has strengths and weaknesses, depending on factors like data complexity and processing costs.
Critically, all these methods require human input—whether it’s defining the number of clusters, setting epsilon distances, establishing minimum points per cluster, or configuring hierarchy metrics. If any of these parameters are off, the AI’s output can be significantly skewed or outright incorrect.
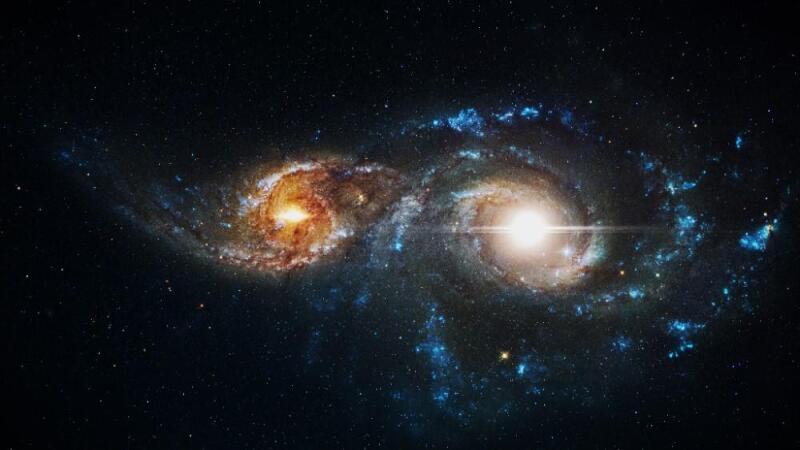
Adobe Stock By alones
AI hallucinations—where LLMs generate false or misleading responses—can stem partly from clustering errors, like misgrouping similar words. Think of a guy joining a sports chat after hearing “shovel pass,” though he knows more about trowels than touchdowns.
Torque Clustering eliminates human oversight, allowing AI to learn and predict patterns autonomously
Supervised learning, which relies on human-labeled data and preset parameters, is costly and inefficient for complex datasets. Torque Clustering removes human oversight, enabling AI to autonomously identify patterns and make predictions more effectively.
Researchers have tested the Torque Clustering algorithm on 1,000 diverse datasets, achieving an AMI (Adjusted Mutual Information) score of 97.7%. This metric evaluates how well data clusters—grouping similar topics like sports with sports and gardening with gardening, even when they share words like “shovel,” “turf,” or “seed.”
In comparison, state-of-the-art clustering methods typically score in the 80% range.
“What makes Torque Clustering unique is its foundation in the physical concept of torque, allowing it to identify clusters autonomously and adapt to various data types with different shapes, densities, and noise levels,” explains Dr. Jie Yang, the study’s first author. “It was inspired by the torque balance in galaxy mergers and is based on two fundamental properties of the universe: mass and distance.”
This approach hints at progress toward artificial general intelligence (AGI), but questions remain. Can AI truly learn without parameters, or are hidden heuristics guiding its path?
The Torque Clustering project—now making headlines—is open-source and available on GitHub. Answers may emerge as more researchers experiment with it. However, since its release in May 2024, it hasn’t seen widespread adoption in AI training, which could already speak volumes.
Read the original article on: New Atlas
Read more: Drone Air Traffic Control Ensures Safe Skies Using Cost-Effective Radars